By Raaka Mukhopadhyay, Aby Tiet, Shambhavi Ramaswamy, Jake Jackson
Background
Virginia House of Delegates District 63 was redistricted in 2019, and Democrats needed to adapt their strategy for the new district in 2021.
Redistricting is the process by which each state redraws new electoral district boundaries. Nationally, it occurs every 10 years in conjunction with the Decennial Census. For each district, the represented populations should be roughly equal in order to ensure equal representation.

Redistricting and gerrymandering have influenced election outcomes for years, and finalized district lines are decided after significant debate and legal proceedings. Already this cycle:
Tennessee state representatives passed a controversial congressional map, which splits up Nashville into three districts;
Ohio’s congressional map proposal was overturned by the Ohio Supreme Court, leading to a prolonged legal and political redistricting process that is still ongoing;
And Maryland recently had to pass a second congressional map after the previous was thrown out by a state judge.
Redistricting is fraught and often flawed, but we are not here to debate that process today. Instead, we are focusing on how you work with the lines you get. Or, in the case of our campaign, how to calculate a win number after redistricting.
Why is calculating a win number after redistricting difficult?
A win number is as simple as it sounds: it is the number of votes a candidate needs to win their election. It is essential to campaign strategy because it helps determine how a candidate spends their limited time and resources.
When our team started working with Lashresce Aird’s campaign for Virginia House of Delegates, we were charged with verifying their calculated win number, a common project that Bluebonnet teams help their campaigns with. As we got started, we referenced different breakdowns of how a typical win number calculation is made, including the formulas below:
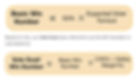
Although a win number calculation usually follows a typical course, after redistricting, the calculation and results are expected to change. For each district, the represented populations should be roughly equal to ensure equal representation. However, partisan leanings and who controls redistricting may skew the district in one direction or another. Districts that in the past would have been a fair fight between two opposing party candidates could result in a heavy swing toward one side.
The above calculations only work if your district is the same year after year. In our case, we were dealing with a variety of complications:
There was only one previous election to base a win number on, based on the data we had available.
And, we had to consider how election trends, such as the influx of mail-in ballots in the 2020 Presidential election, might impact the 2021 election.
How We Calculated Our Win Number
When you don’t have the “typical” information to calculate the win number, it is acceptable to make changes to the typical formula and improvise using the information you do have. After reviewing the information available with our campaign, we made changes to the expected votes formula and identified an adjustment percentage. We can summarize our changes to the formula as such:

We began by compiling a database of demographic information for the district. We’ll go over what numbers we used and why in the latter portion of this post.
Then, we looked back at the most recent election data from 2019. To calculate expected ballots, we averaged 2019 turnout from three different sources: VoteBuilder, VPAP, and internal data from our campaign. For our district, since we had less information from prior election cycles, we chose to present our calculation in a range, allowing our calculation to be more accurate within the scope of potential election outcomes. We calculated the expected votes cast with a 4-5% adjustment percentage (which is part of the Expected Votes Cast equation from above) by discussing with our campaign manager what seemed like an appropriate estimate.
Finally, we calculated the win number using both a 1% and 2% safety margin, which is part of the Win Number equation. We considered the safety margin especially important for our campaign because the district data in VoteBuilder was not as up-to-date as we would have liked it to be — we wanted to provide an additional safety net yet avoid overcompensating.
Our calculations led us to the following result: we predicted that the campaign gaining between 12,893 to 13,189 votes would lead to success for the Aird campaign with a 51% margin. Prior to joining the campaign, they had calculated a win number similar to ours. However, when the election was said and done, even with 16,301 votes, Lashrecse had lost her campaign by a margin of 512 votes. Although we trusted our calculation, it’s important to remember that predictions are, simply, predictions. We did try to account for changes in redistricting, but our teams’ prediction was off by several thousands votes. In the next section, we dive into lessons learned for how to predict a more accurate win number despite the significant challenges presented by redistricting. In this retrospective analysis, we were able to identify the core likely missing factors in our analysis and revise our original calculation to reach an accurate win number estimate.

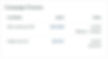
Learned Lessons for Future Bluebonnet Teams
In retrospect, one of our biggest limitations was data sourcing. The challenge of campaign data analytics is so often being able to source the most comprehensive and accurate data from which to create insights. For our original analysis, we focused on 3 estimates for 2019 turnout data. For future analyses, we would look to source data that is more comprehensive by including more past election cycles and accurate by sourcing directly from the Virginia Secretary of State reported vote totals.
Making our Data more Comprehensive:
We used 2019 election turnout as an indicator for 2021 election turnout. However, this data is not fully comprehensive of the political context leading into the 2021 election, primarily because 2021 was a Gubernatorial election year while 2019 was not. Though we did not use data from a Gubernatorial election, we did take this factor into consideration when calculating the win number; nonetheless, we can still provide a more data-informed estimate. The chart below illustrates how Gubernatorial state election years have significantly higher voter turnout because high-profile, statewide campaigns for Governor can draw more attention and enthusiasm from voters than purely local election campaigns in Non-Gubernatorial state election years like 2019. Factoring in increased Gubernatorial turnout would raise our win number estimate by approximately 12%.

Another trend that this more comprehensive dataset illustrates is a long-term trend of increasing voter turnout, perhaps due to a nationwide increase in political polarization and engagement over the past decade. Factoring in overall year-over-year increasing turnout would raise our win number estimate by approximately 11%.
Making our Data more Accurate:
In our original analysis, we relied on 3 sources for estimates of past turnout data: Votebuilder (VAN), VPAP, and internal campaign data. In retrospect, we determined that the use of VAN led to a potential undercount of past vote totals since VAN excludes people who have since moved out of the district or passed away. VPAP and internal campaign data estimates of past turnout likely weren’t undercounted like VAN, but may still have been less precise due to the difficulties of aggregating past election data into new district lines. Ultimately, election history data is best sourced–whenever possible–directly from the Secretary of State (or Department of Elections, etc) in that state. With redistricting, it is harder to aggregate this data into new district lines but still possible by using precinct-level data where available. Factoring in an undercount of past turnout data by using Votebuilder in our original analysis would raise our win number estimate by approximately 4%.
Combining all of these factors not included in our original analysis raises our original win number calculation by 29%, for a calculated win number of 16,850 votes, which is essentially exactly how many votes the Republican won with in 2021.
While our original calculation was a slight undercount, it is worth noting that it was still more accurate than the campaign’s internal calculations prior to our team’s analysis. Furthermore, by sharing this retrospective analysis, we are able to better inform future Bluebonnet Data & other campaign data analytics teams in tackling similar projects throughout the 2022 election cycle, where the overwhelming majority of campaigns across the country will be dealing with newly-drawn district lines.
Other advice we want to provide specific to Bluebonnet teams are:
Before getting started, take a look at your area’s Census data to get an idea of the population, including education, income, racial, and gender metrics.
Check if there are other databases (for example in VA, there’s VPAP) that provide election results breakdowns by locality and precinct for previous years. For turnout data, be sure to source data directly from the Secretary of State’s office. Typically, precinct-level election history data is available and can be aggregated into new district lines.
Ask in the Bluebonnet Forum to see if anyone else is looking for similar data or dealing with similar issues.
Reach out to us, Bluebonnet mentors (shoutout to Caiseen!), or other experienced teams for additional tips to improve your calculation
About the Authors
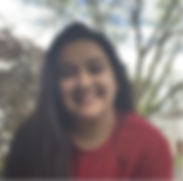
Raaka Mukhopadhyay was a Data Fellow for Lashresce Aird’s campaign for Virginia State House in District 63. She is majoring in Statistics and Machine Learning at Carnegie Mellon University
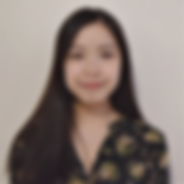
Abigail Tiet was a Data Fellow for Lashresce Aird’s campaign for Virginia State House in District 63. She is majoring in Software Engineering and minoring in Political Science at Rochester Institute of Technology.
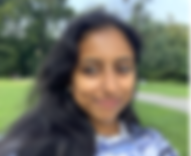
Shambhavi Ramaswamy was a Data Fellow for Lashresce Aird’s campaign for Virginia State House in District 63. She is majoring in Computer Science and Economics at Rutgers University.
If you like what you’ve read and want to learn more, you can reach out at info@bluebonnetdata.org. Or, If you're interested in doing similar work, apply to be a Data Fellow!